Testifying for H.2701 in the MA Legislature: a committee to audit government use of AI systems
Yesterday, I testified in the MA legislature in support of H.2701 and S.1876, bills that look like a strong and important first step towards ethical and careful use of algorithms and AI in governance. It’s a little sad, but this “radical first step” is simply to create a commission that collects information on where automated decision systems are being used. That’s it. This sounds simple, but collecting any centralized info about what a state government is doing is a pretty rare thing.
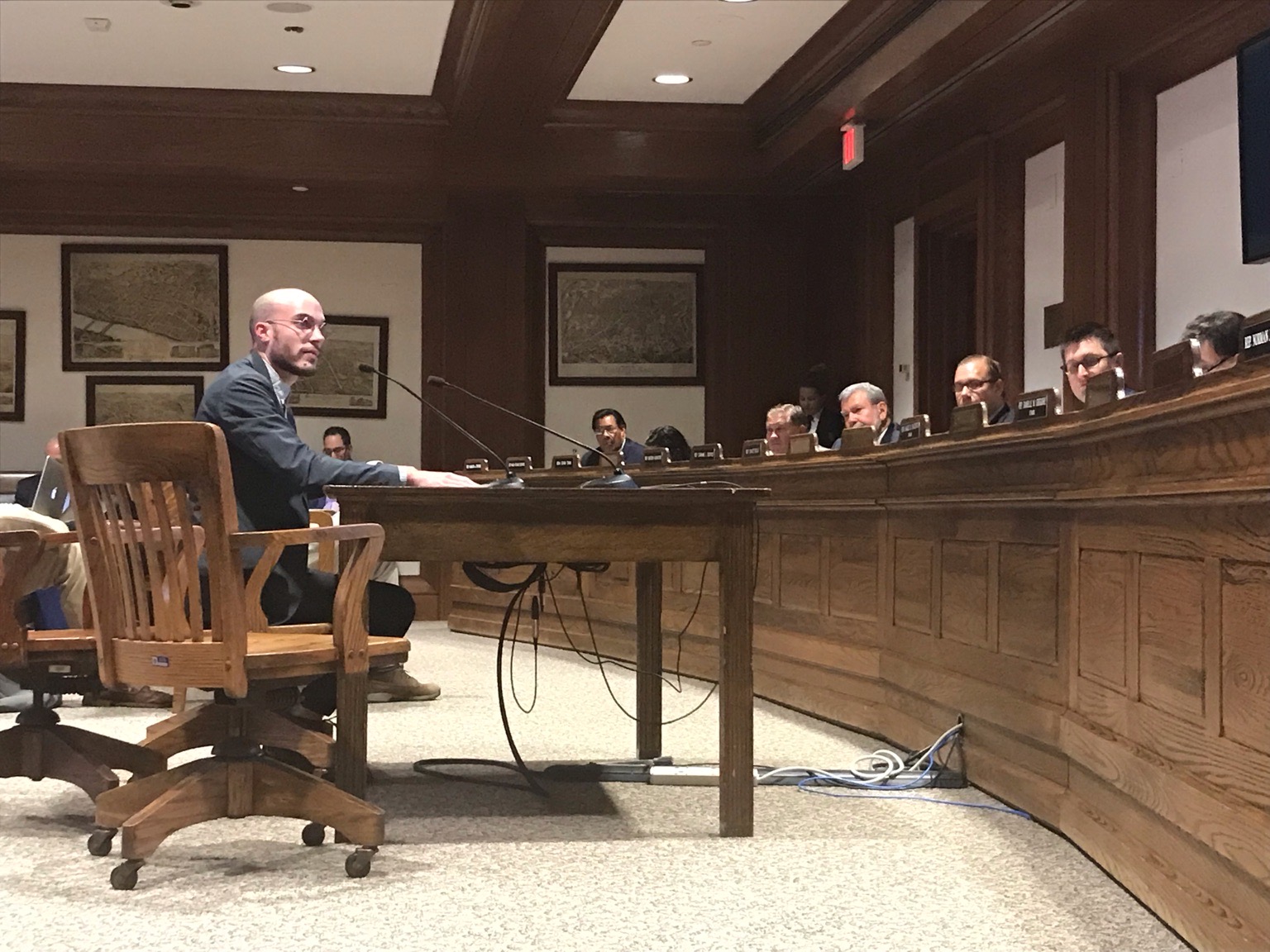
I got the opportunity to testify alongside Suffolk Law Professor Gabriel Teninbaum, EPIC Policy Director Caitriona Fitzgerald, MIT Researcher Karthik Dinakar, and ACLU’s incredible Kade Crockford, among others. You can read my full written testimony below:
Support for SB.1876 & H.2701: a crucial step in ensuring fair future governance in the commonwealth.
Dear Senator Pacheco, Representative Gregoire, and members of the Committee:
I’m writing in strong support of S.1876 and H.2701, An Act Establishing A Commission On Transparency And Use of Artificial Intelligence In Government Decision-Making and An Act Establishing A Commission On Automated Decision-Making, Artificial Intelligence, Transparency, Fairness, And Individual Rights. To shape the future of how we use artificial intelligence and other technologies in governance, we need to understand how they are being used in governing systems right now. This legislation will provide a desperately needed avenue for policymakers, researchers, and the public to understand how algorithms and artificial intelligence are impacting government decision-making and constituents.
As a doctoral researcher studying data governance and machine learning at MIT, I have experience working with AI tools and reasoning about fairness and ethics in how they are used. My research experience includes developing cutting-edge machine learning systems, designing smartphone apps that use AI to better understand and prevent chronic disease, measuring segregation in cities using machine learning and big data, and writing about the ethics of collecting large amounts of data about citizen’s behavior, such as their location history, for use in governance. I have had the opportunity to present work on these topics at international conferences on machine learning, social science, and data ethics. My unique research experience has given me expertise on the implications of using algorithms in governance, including its promises and pitfalls, how data is collected from the public to fuel these algorithms, and how these algorithms function.
As it is used today, artificial intelligence or automated decision-making mainly refers to the use of computer programs that can make predictions or provide insights from data. Many AI tools are currently commonly used by governments in the U.S. Specific examples include the automatic creation of pre-trial risk scores in the justice system using data about a defendant, using facial recognition algorithms to identify people automatically using images of their face, and even using AI to process social media data, like Facebook posts or Tweets to predict civic unrest or protests.
The main issue this proposal addresses is that algorithms without oversight are being used to make decisions in governance today, and their use will only increase.
A core problem that this legislation addresses is that while such algorithms are currently used in making decisions across the country and here in Massachusetts, there are no laws that require any transparency or accountability regarding their use. While experimenting with new forms of governance and decision-making that may provide benefits to the public is good, such experiments need to be made clear to citizens and to members of government in order to protect basic civic liberties.
Many people think that decisions made by algorithms are always correct because they are made by a machine. But algorithms, like people, make biased or incorrect judgments and decisions all the time. For example, it is well known that many facial recognition algorithms are better at recognizing white people’s faces than the faces of people of color. This becomes an enormous issue when used in governance. Using this algorithm could mean longer wait times at the RMV for an entire group of people, but it can also mean identifying the wrong person as the perpetrator of a crime, having disastrous implications for civil liberties and people’s livelihoods.
There is an entire research field on Algorithmic Fairness which exists because thousands of researchers, including me and those in my lab, agree that how algorithms impact people is a pressing, urgent challenge in modern society. It is possible to measure how fair or biased an algorithm is in specific contexts, but only if the algorithm is accessible, if researchers and policymakers have access to reports on the algorithm, and if the data used to “train” the algorithm is made available for scrutiny. It is also possible to “explain” how some algorithms arrived at a decision, but only if enough information about the algorithm can be shared. Ensuring that algorithms can be used to help good governance can only happen with sufficient transparency and accountability.
These tools have implications beyond the specific decisions being made as well. In order to “train” algorithms to make decisions, they must be given what is called “training data”: examples of how decisions have been made in the past. Two important problems appear when this training happens without oversight. First, algorithms generally need many, many examples to be fully trained, so to train an algorithm, an agency must collect and store a large amount of data about its constituency. This can have enormous privacy implications depending on the data being stored. For example, a transit agency might purchase data from Lyft or Uber on how individuals move around a city in order to better plan new transit lines. Without proper data governance structures, this data could easily be used by state employees to identify particular individuals for personal interest or gain, or used in ways that individuals do not consent to. There are solutions to this problem that my research lab and others have worked on, but they cannot be deployed or even considered without understanding what kind of data is being collected and aggregated, where, and for what purpose.
The proposed bill: create a commission to document where automated decision systems are being used, and recommend guidelines for how they should be used in the future to protect individuals and communities.
The core of the proposed bill is to create a commission that will leverage local expertise of data and algorithmic systems to recommend guidelines for how automated decision systems and algorithms should be used throughout the Massachusetts government. One of its main outcomes is a collection of information about the current use of algorithms and automated systems in government, and their speculated impact on citizens. This is crucial for developing accountable and democratic governmental systems that are consistent with the Commonwealth’s values.
First, to protect individual civil liberties, we must be able to audit and examine existing and future uses of algorithms in government decision-making. Algorithms and automated decision systems can be helpful, but they often provide information that is biased, or make decisions that are unfair. This bill is a first step towards ensuring that every person has access to equal services and fair trials by potentially allowing government agencies, watchdog groups, and citizens to understand where automated decision systems are used.
Second, increased transparency will help various stakeholders in the commonwealth contribute to more effective governance. This bill will create possibilities for future laws and policies that could allow people to appeal decisions that may be deemed unfair, give experts and agencies opportunities to assess and improve the systems’ usefulness and impact, and inform a public discussion on where and when it is appropriate to use algorithms in government decision-making at all. To design future systems that improve governance and treat all citizens of the Commonwealth equally, we must allow citizens and stakeholders to examine how automated decisions in government are being made.
Third, many government decision systems are provided by third parties and use large amounts of personal data collected about citizens. This creates new risks for individual liberties and raises questions about data ownership and rights. The risk of personal data being abused by state employees, or obtained through unauthorized access, is real. While there are solutions that can help mitigate this risk and establish citizen’s rights to their personal data, it is impossible to test them without transparency into how data is being collected or purchased, how it is being used and stored, and what systems it is being used for.
The proposed legislation will create a commission, informed by experts in the field, that will perform an initial overview and analysis of where automated systems are being used the Commonwealth. Their analysis will include identifying where using these systems may put individual liberties and welfare at risk, an important step in producing the commission’s final products: recommendations on how to ensure that these systems avoid harming individuals and communities, and, crucially, a report to the general public detailing current uses of automated decision systems and algorithms in governance that impacts citizen’s welfare.
As a researcher with knowledge of how these systems operate and how their use may impact society generally, I strongly support this legislation. I believe it is an important first step towards developing systems that can help us achieve better governance, and that without it, we will end up with governing structures that do not reflect the Commonwealth’s values. I recommend the committee to quickly report favorably on S.1876 and H.2701.
Sincerely,
Dan Calacci
Doctoral Student, MIT Media Lab